WebQA 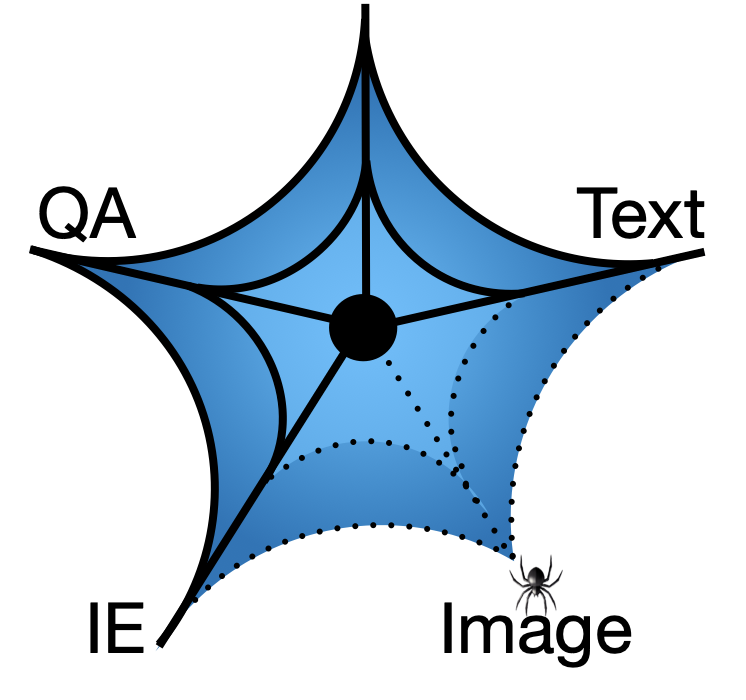
Yingshan Chang | Yonatan Bisk | Mridu Narang |
Guihong Cao | Hisami Suzuki | Jianfeng Gao |
WebQA, is a new benchmark for multimodal multihop reasoning in which systems are presented with the same style of data as humans when searching the web: Snippets and Images. The system must then identify which information is relevant across modalities and combine it with reasoning to answer the query. Systems will be evaluated on both the correctness of their answers and their sources.
Task Formulation: Given a question Q, and a list of sources S = {s1, s2, ...}, a system must a) identify the sources from which to derive the answer, and b) generate an answer as a complete sentence. Note, each source s can be either a snippet or an image with a caption. A caption is necessary to accompany an image because object names or geographic information are not usually written on the image itself, but they serve as critical links between entities mentioned in the question and the visual entities.
Evaluation: Source retrieval will be evaluted by F1. The answer quality will be measured by Fluency (BARTScore) and Accuracy (keywords overlap).
For more questions and communication please subscribe to our mailing list!News: NeurIPS Challenge winners! 🎉 🎉 🎉
1st place: VitaminC (Ping An)
2nd place: Soonhwan Kwon,Jaesun Shin (Samsung SDS AI Research)
3rd place: Intel Labs Cognitive AI and MSRA NLC
Paper on ArXiv» Data Download» Leaderboard» Baseline Code» Blog»
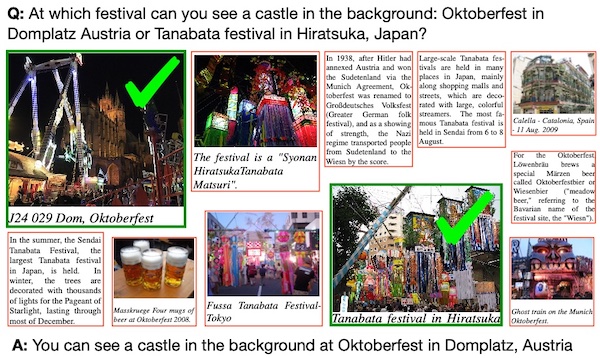
@inproceedings{WebQA21,
title ={{WebQA: Multihop and Multimodal QA}},
author={Yinghsan Chang and Mridu Narang and
Hisami Suzuki and Guihong Cao and
Jianfeng Gao and Yonatan Bisk},
journal = {ArXiv},
year = {2021},
url = {https://arxiv.org/abs/2109.00590}
}